A Recent UMMA Study Shows What Happens When You Use Facial Detection Software to Analyze an Art Museum’s Collection
Results offer a warning to those developing and using the technology, prompts more critical questions about fair representation
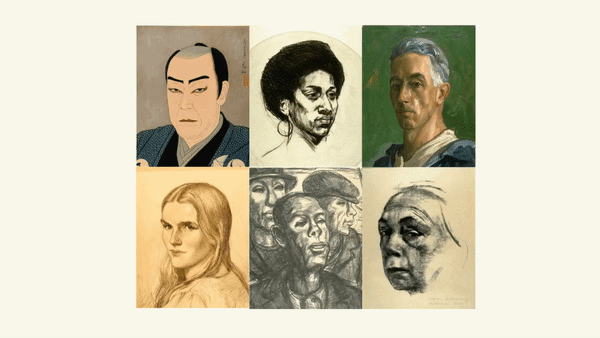
Animated image by Shannon Yeung (BFA ’22)
A team of U-M researchers, including 2 UMMA staff members, recently released the results of a year-long study that used the Museum’s collection to investigate questions about machine learning and representation in the arts. For the study, researchers used existing artificial intelligence algorithms — which have been trained to recognize facial features and the racial and gender demographics of faces — to analyze the works of art UMMA has collected over the past 150 years.
“Our research was both trying to further understand the apparent diversity of the Museum’s collection with respect to the human faces present in the art, while also surfacing the dangers of using algorithms trained on limited data sets to analyze other collections of data,” said John Turner, UMMA’s Senior Manager of Museum Technology, who was part of the team.
Facial detection software, which is simply an algorithm deciding whether or not a human face exists in an image, is not quite as advanced as facial recognition software, which attempts to identify who the face belongs to. Both have a variety of uses, from the benign to the controversial–for example, facial detection is used when a self-driving car detects that a pedestrian is up ahead, and facial recognition is used by law enforcement officials worldwide when examining security footage of crimes.
”I thought it would be really interesting to see how accurate a first pass at using this technology in a museum collection setting might be,” said Turner. “There has been so much promise and hope placed in AI/Machine learning to help assist collections managers and researchers to more deeply and accurately catalog, describe, and analyze visual resource collections, but there is clearly still a long way to go to leverage these technologies in a practical way at scale.”
Our research was both trying to further understand the apparent diversity of the Museum’s collection with respect to the human faces present in the art, while also surfacing the dangers of using algorithms trained on limited data sets to analyze other collections of data.
John Turner, UMMA’s Senior Manager of Museum Technology
The researchers noted that the face detection algorithm had mixed success with UMMA’s collection. In some cases, it was able to detect faces that were small or abstract, not only those that were straightforwardly depicted in portraits. In other cases though, it “detected” faces that did not exist (such as finding a face in the light reflecting off of a still-life pear, or in the bumpy sides of pottery), and failed to find faces that would have been obvious to a human viewer. For unknown reasons, the algorithm had a particularly tough time finding faces in woodblock prints and woodcuts.
After first just detecting if a face was present, the researchers then used a race classification algorithm (based on a dataset of photos from Flickr) in order to look at the apparent racial diversity present in the Museum’s collection. Once again, an algorithmic approach to this type of analysis had important shortcomings and complications that impacted what and how the code was able to classify images. For instance, the source images used to train the algorithm lacked Native American faces, so the portraits of Native Americans in UMMA’s collection were classified as a variety of other races.
Overall, researchers determined that UMMA’s collection has become more diverse over time and that, among art featuring human faces, the percentage of art featuring non-white faces has increased since the early 1900s. Also, the researchers noted a few anomalies that lead to misleading interpretations–such as a finding that all acquisitions in 1919 supposedly included Black faces. In fact, however, there were only 3 acquisitions that year, and all were ones in which the algorithm detected human faces when there were none actually present.
![]() |
Photo by Marc-Grégor Campredon |
Turner noted that the algorithm’s lack of accuracy was somewhat expected, and part of the purpose of the project. “We weren't seeking to correct the algorithm or train a new one,” he said. “We merely wanted to test the performance and accuracy of existing algorithms already in use elsewhere to see how well they stack up against reality and to have their failings serve as a warning that people should think critically about the application of these types of algorithms given the bias that might be present in their creation and interpretation.”
The research culminated in two videos called “White Cube, Black Box” (put together by Shannon Yeung, BFA ’22) which are currently playing side-by-side in UMMA’s Lizzie and Jonathan Tisch Apse.
The first video gives context for how the researchers undertook the project. “The phrase ‘White Cube’ is a term that refers to museums historically being exclusionary and having blank white walls and removing all the context, which makes the work actually quite inaccessible for those who aren’t highly educated in the subject matter or coming from certain communities,” explained Stamps Associate Professor Sophia Brueckner, who was one of the principal investigators. “And, the term ‘Black Box’ is used in engineering to talk about how a lot of these technologies that we rely on are opaque.”
In addition to Brueckner and Turner, the rest of the team of researchers included Dave Choberka (Andrew W. Mellon Curator for University Learning and Programs at UMMA), Jing Liu (Managing Director of the Michigan Institute for Data Science), and Kerby Shedden (Center Director at Statistical Consultation and Research).
The videos for White Cube, Black Box are available to view in UMMA’s You Are Here exhibition, as well as on Youtube.
Fair Representation in Arts and Data: White Cube Black Box 1
Fair Representation in Arts and Data: White Cube Black Box 2
White Cube, Black Box is supported by the University of Michigan Arts Initiative.